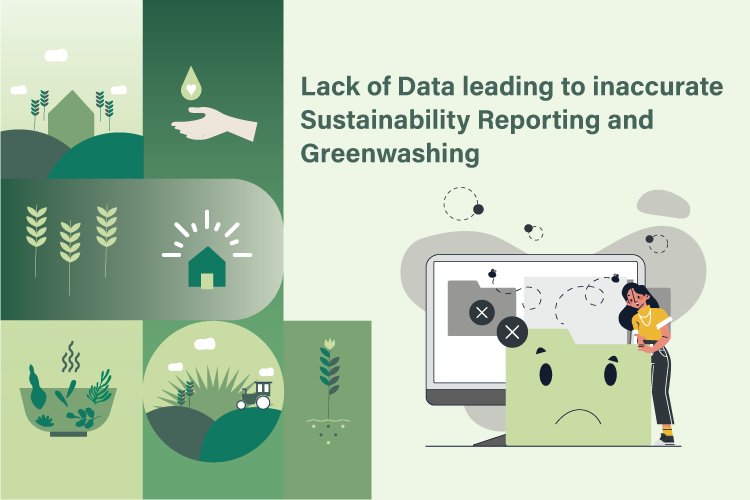
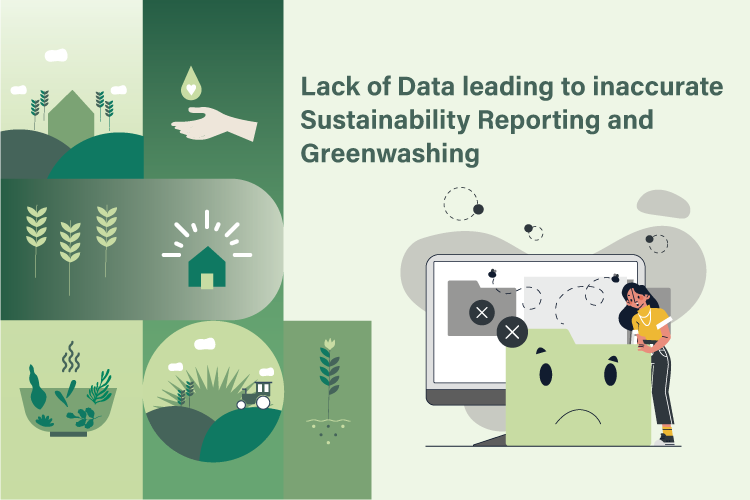
Lack of Data leading to inaccurate Sustainability Reporting and Greenwashing
The biggest challenge in implementing sustainability in any business is the lack of data. While we see that every business process is automated, we find that the data is not clean. There is lot of duplication, data is fragmented. Lot of data is stored from very old days and is not relevant anymore. All these are leading to Sustainability Reporting to be more guesses and aspirations.
Some of the statistics on Data:
Data Fragmentation:
According to the World Economic Forum, over 70% of companies consider data fragmentation as a major challenge in sustainability reporting. This can make it difficult to gather comprehensive data on environmental and social impacts.
Data Quality and Reliability:
The World Business Council for Sustainable Development reports that more than 30% of sustainability data is unreliable or of poor quality, undermining the credibility of sustainability initiatives.
Data Volume:
The amount of data generated on environmental, social, and governance (ESG) factors is growing rapidly. According to the Global e-Sustainability Initiative, in 2021, the world produced around 59 zettabytes (1 zettabyte = 1 trillion gigabytes) of data, much of which relates to sustainability.
Data Collection Costs:
Gathering high-quality sustainability data can be expensive. The United Nations Development Programme (UNDP) estimates that for many countries, the cost of collecting and managing environmental data can be as high as 1-2% of GDP.
Data Accessibility:
A significant portion of sustainability-related data is not publicly available. In fact, the UN Environment Programme found that only 6% of the required data is accessible in the public domain.
Lack of Standardized Reporting:
According to a report by CDP (formerly the Carbon Disclosure Project), in 2020, only 9% of the world’s 500 largest companies reported on all environmental key performance indicators (KPIs) related to their activities.
Incomplete and Inaccurate Data:
A study published in the journal Nature Communications in 2020 found that companies often underreport their carbon emissions, making it challenging to assess their true environmental impact.
Limited Access to Supply Chain Data:
According to a 2019 report by CDP, 61% of greenhouse gas emissions from major corporations are associated with the supply chain.
Consumer Awareness:
A 2020 survey by Ipsos found that 42% of consumers had encountered products or companies that they suspected of greenwashing.
Sustainability and the data problem leading to greenwashing are complex topics in the context of sustainable investing and corporate responsibility. Let’s break down these concepts and their interplay in detail:
- ESG (Environmental, Social, and Governance):
ESG refers to a set of criteria used by investors, analysts, and organizations to evaluate a company’s performance and impact in three key areas:
- Environmental: This category assesses a company’s impact on the environment, including its carbon footprint, water usage, waste management, and efforts to reduce pollution and resource consumption.
- Social: The social aspect looks at how a company treats its employees, customers, suppliers, and communities. It includes factors like labour practices, employee diversity and inclusion, product safety, and community engagement.
- Governance: Governance evaluates a company’s management structure, board composition, shareholder rights, and ethical business practices. It aims to ensure transparency, accountability, and responsible decision-making within the organization.
- Greenwashing:
Greenwashing occurs when a company or organization exaggerates or misrepresents its environmental or social commitments and practices to appear more environmentally or socially responsible than it actually is. This can mislead investors, consumers, and the public, making them believe that the company is more sustainable and ethical than it truly is.
- The Data Problem Leading to Greenwashing:
The data problem associated with Sustainability and greenwashing arises from several challenges:
- Lack of Standardization: There is no universal framework or set of metrics for Sustainability reporting. Companies often report Sustainability data using different standards and metrics, making it difficult for investors to compare and assess their performance accurately.
- Data Quality and Reliability: Sustainability data can be incomplete, inconsistent, or unreliable. Companies may not disclose negative information or may engage in selective reporting, highlighting their positive efforts while downplaying their negative impacts.
- Scope and Materiality: Determining which Sustainability factors are most material to a particular industry or company can be subjective. Some companies may prioritize less significant Sustainability factors to present a positive image while neglecting more critical issues.
- Limited Regulatory Oversight: There is limited regulatory oversight and enforcement in Sustainability reporting, leading to a lack of accountability for companies engaging in greenwashing practices.
- Complexity of Sustainability Issues: Sustainability issues are multifaceted and interrelated, making it challenging to capture their full impact accurately. For example, a company may reduce its carbon emissions but may still have problematic labour practices.
Let us see How Greenwashing Occurs due to Data Problems:
- Misleading Metrics: Companies may cherry-pick Sustainability metrics that present them in a favourable light while downplaying negative aspects.
- Inadequate Disclosure: Companies may not fully disclose relevant Sustainability information, making it difficult for investors and stakeholders to assess their true performance.
- Lack of Verification: Without standardized and independently verified Sustainability data, investors may rely on self-reported information, which can be unreliable.
- Complexity Masking: Companies may focus on one Sustainability dimension to divert attention from other, more significant Sustainability issues.
- Limited Transparency: Lack of transparency can hide a company’s actual Sustainability practices and commitments. Scope 2 and Scope 3 Emission data is difficult to get. Scope 3 data needs to come from the Supply Chain, both upstream and downstream. There is no obligation for anyone to provide the data, cutting across departments, organizations, domain and geographies, thus making it more difficult to get the data.
Greenwashing is a significant problem because it can mislead consumers and hinder genuine efforts to address environmental issues. To combat greenwashing and address the data problem, efforts are underway to standardize Sustainability reporting frameworks, increase transparency, and promote independent verification of Sustainability data. Investors, regulators, and organizations are working together to establish more rigorous standards and practices to ensure that Sustainability investments and commitments align with true sustainability goals.
- Role of Information Technology in solving the data problems:
By providing transparency, accurate measurement, and verification of environmental claims IT can help:
- Data Collection and Monitoring:
IoT (Internet of Things) Sensors: IoT devices can be deployed to collect real-time data on environmental factors like energy consumption, emissions, water usage, and waste production.
Remote Sensing: IT can enable the use of satellite imagery and drones to monitor deforestation, pollution, and other environmental indicators.
Data Integration: IT systems can consolidate data from various sources, including sensors, databases, and external datasets, to create a comprehensive view of environmental impacts. We see ERP provider like SAP adding many features to capture Sustainability information at the business process level, along with separate portal to collect data from the Supply Chain providers.
- Data Analysis and Reporting:
Big Data Analytics: IT tools can process large volumes of data to identify patterns, trends, and anomalies in environmental data.
Machine Learning and AI: These technologies can help predict environmental impacts and provide insights for sustainable practices. There should be ethical and responsible use of these. We must see that the positive sustainability impact is significantly more than the negative sustainability impact.
Visualization: IT can create interactive dashboards and visualizations to communicate environmental data effectively to stakeholders and consumers.
- Blockchain for Transparency:
Blockchain technology can be used to create immutable and transparent records of environmental data. This can ensure that data related to carbon emissions, supply chain sustainability, and other environmental metrics are tamper-proof and verifiable. Tracking the Supply Chain will benefit a lot from the use of Blockchain technology use.
Smart Contracts: Blockchain can automate agreements and transactions based on environmental performance, making it difficult for companies to make false claims.
- Standards and Certifications:
IT can support the development and implementation of standardized reporting frameworks like Global Reporting Initiative (GRI) and Sustainability Accounting Standards Board (SASB).
Certification authorities can use IT to verify and validate environmental claims made by companies. Blockchain and cryptographic techniques can enhance the trustworthiness of such certifications.
- Crowdsourcing and Transparency Platforms:
IT platforms can enable crowdsourced environmental data collection and reporting, allowing consumers and NGOs to contribute to the verification process. IT can help in building an ecosystem of data.
Transparency platforms can provide easy access to environmental data for consumers, making it easier to verify claims and make informed choices.
- Regulatory Compliance:
IT systems can assist regulatory authorities in monitoring and enforcing environmental regulations by automating data collection, reporting, and compliance checks.
Compliance data can be stored securely and shared transparently through blockchain technology.
- Consumer Education:
Mobile apps and websites can provide consumers with tools to scan product barcodes and access detailed information about a product’s environmental impact, helping them make informed purchasing decisions.
- Auditing and Verification:
AI and machine learning algorithms can be used to detect anomalies or discrepancies in reported environmental data, triggering audits when necessary.
Third-party auditing firms can use IT to conduct more efficient and accurate assessments of a company’s environmental claims.
Information Technology can play a pivotal role in addressing the data problems that lead to greenwashing by improving data collection, analysis, transparency, and verification. By leveraging IT solutions, companies can provide accurate and reliable information about their environmental performance, which is essential for promoting genuine sustainability efforts and holding those who are greenwashing accountable. However, this requires ethical and responsible use of Technology, good data governance and above all a sense of Trust among the Ecosystem Stakeholders.
- How DataOps can help:
On day-to-day operations of an organization practicing DataOps will be very helpful. Data has to be given significant focus and treated as first class citizen.
DataOps is a set of practices and principles aimed at improving the collaboration, communication, and automation within the data management and analytics process. It helps organizations streamline their data pipelines, ensure data quality, and make data-driven decisions efficiently. When it comes to addressing data problems that can lead to greenwashing, DataOps can play a crucial role in promoting transparency and accountability in sustainability reporting in following ways.
- Data Collection and Integration:
Problem: Greenwashing often occurs when organizations selectively collect or manipulate data to create a favourable impression of their environmental impact.
DataOps Solution: DataOps promotes a systematic and standardized approach to data collection. It ensures that all relevant data sources are integrated, and data is collected consistently and comprehensively. Automation can be used to gather data from various sources in real-time, reducing the likelihood of data manipulation.
- Data Quality Assurance:
Problem: Inaccurate or incomplete data can lead to misleading sustainability reports, contributing to greenwashing.
DataOps Solution: Data quality is a fundamental aspect of DataOps. It involves data profiling, cleansing, and validation processes to identify and rectify data errors. DataOps also emphasizes data lineage, making it clear where data comes from and how it’s transformed, enhancing transparency
- Data Governance:
Problem: Greenwashing can occur when there is a lack of data governance, leading to data being used inappropriately or without proper authorization.
DataOps Solution: Data governance frameworks are an integral part of DataOps. They define policies and procedures for data access, usage, and security. This ensures that only authorized individuals can access and manipulate data, reducing the risk of data misuse.
- Data Transparency:
Problem: Greenwashing often thrives in environments with limited data transparency.
DataOps Solution: DataOps promotes transparency by providing data catalogues and metadata management. Users can easily access information about data sources, definitions, and transformations, making it clear how sustainability metrics are calculated.
- Data Auditing and Monitoring:
Problem: Without ongoing monitoring, data discrepancies and manipulation can go undetected.
DataOps Solution: DataOps includes robust auditing and monitoring capabilities. Automated alerts and notifications can be set up to notify stakeholders when data anomalies or discrepancies are detected. Regular audits ensure data accuracy and credibility.
- Collaboration and Accountability:
Problem: Greenwashing often occurs when different departments within an organization operate in silos, leading to inconsistent data reporting.
DataOps Solution: DataOps fosters collaboration by breaking down silos. Cross-functional teams work together on data projects, ensuring that sustainability data is consistent and reliable. Accountability is enhanced as individuals and teams take ownership of data pipelines and processes.
- Continuous Improvement:
Problem: Greenwashing can persist if data processes remain static and are not continuously improved.
DataOps Solution: DataOps encourages a culture of continuous improvement. Metrics are tracked, and feedback loops are established to iteratively enhance data collection, processing, and reporting processes.
By implementing DataOps practices, organizations can ensure that their sustainability data is accurate, reliable, and trustworthy, reducing the risk of greenwashing and enhancing their environmental credibility.
We thus see the importance of data, the current problem with data availability, data accessibility, data cleanliness, data security and data governance which is required for sustainable business. We need to build the ecosystem, a widespread Data Platform and Trust among the Stakeholders of the ecosystem to enable better and accurate sustainability reporting.
Author: Dr. Niladri Choudhuri